A short history of Data
Tons of data are produced at different enterprise levels every day. Many different ways exist to classify data. There are operational data as well as analytical data, structured and unstructured data, internal and external data, actual and metadata.
On operational level one can find transactions, transactional states, transaction Metadata, reconciliation data, master data, pricing and calculation data, data about operational risks and customer classification. Last but not least there are a lot of business partner communications over different channels in several unstructured formats.
On analytical level many enterprises have created data warehouses and data marts during the past 20 years. The first and foremost target of those early analytical data stores was to support a comprehensive management information system by creating flexible reporting facilities.
Data Mining came into the play a few years later. Techniques such as automatic generation of decision trees with entropy measures like GINI index, clustering algorithms, found their ways into data analysis in the early days of data mining.
One big issue with data ware houses has always been the data quality and data enrichment. This is where data cleansing methods were introduced. External data sources have been used to enrich data or to get higher quality. But this was not the only intention of adding external data sources into the system. Enrichment can be used to get more data related to a subject and to correlate them with the existing data in order to achieve new insights.
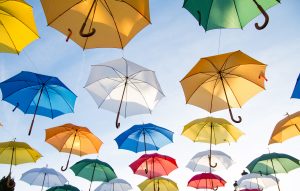
The Rise of Big Data
As technology evolved and new methods of handling and analyzing any kind of data appeared the value of data as an economic property increased. Big data, data science and artificial intelligence brought new opportunities to create Knowledge from Data. To relate different kind of data to each other in ways they haven’t been related before came into the spotlight.
With those new capabilities new type of data became more interesting for analytics purposes. Data about data so called Metadata which one can imagine of as the data definition or data access statistics on the one hand side and unstructured external data such as content of social media sources like facebook, twitter, etc. on the other side.
Many topics like data extraction, transformation and loading, data cleansing and enrichment, data protection and data privacy one will face on technical level in big data initiatives have been in place in data warehouse implementation already. Others like handling streaming architectures, automatic data type recognition emerged as specific topics to Big Data.
The organizational challenges are much bigger in big data since big data has a more open scope than the very specific scope of Data warehouses.
Data Strategy, what’s that?
Data become strategic and hence there is a need for a systematic approach both on business as well as on technical level to improve value created by data.
On business level an enterprise need to define
- what should be achieved with data (vison),
- which data are needed for that goal,
- which methods are they going to apply to create new insight from that data and
- what income that knowledge can lead to?
This is what a data driven business model is about. It stands at the very beginning of a data strategy an enterprise has to develop and follow in terms of monetarizing data.
A data driven business model can be thought of the same way one creates a common business model. Imagine a
business canvas. There you have a rectangle for key resources a business needs to create a value proposition. Data are such key resources. Hence, a data driven business model must give answers to the same questions asked in a business canvas.
Coming from a business canvas approach it is also necessary to define a set of key activities to be taken in order to create the value proposition. What is an enterprise going to do with the data? Which kind of analysis methods should be applied in order to create information which leads to that knowledge? By finishing this task the most important part of a data strategy will be already delivered.
Causation vs. Effectuation
When it comes to choosing the right analysis methods for the data it is important to know the kind of problem that exists. In decision making there are two different classes of problems.
In the first one there is a given predictable effect with a known probability. The target is to choose information gathering and analysis methods to select between the means to create that effect. This is called causation.
In the second there are several unpredictable effects in place. The target is to find out which effect is more likely to emerge with given means. In this case the analysis has to apply experimental techniques to discover the underlying distribution of the unpredictable effects for given means.
In data analysis one might follow a hybrid approach where both analysis methods can be applied.
Different Data Strategy approaches from here
During getting deeper into this subject I have discovered different definitions of a data driven business model. Some experts require a data strategy should also define a project plan which describes how an actual subset of the data should be analyzed with milestones, budget and all that project management stuff we all know.
Other experts of data strategy development stop with creating a data driven business model.
One
interesting approach I have recognized starts with definition of key actors and key data. Then it switches to the customer and creates a customer profile. Value proposition is created by a so called Data-Need-Fit. From value proposition other parts of the business model such as key activities are derived.
But ….
Experts always call out the need for a systematic approach when a new idea and method appears. I have seen a lot of them in the past. Well prepared and best sold systematic approaches to master new challenges.
At the end of the day one can measure the actual value contributed to the enterprises success by the impact an initiative has to the enterprises revenue regardless of how systematic and well prepared that initiative was.
Unfortunately many of those approaches end up helping their inventors to create value.
If you want to have an advice, choose the people for implementing your data strategy wisely.
That’s the most important thing.
For further reading …
Data and Analytics – Data-Driven Business Models: A Blueprint for Innovation
The new hero of big data and analytics: The Chief Data Officer
Effectuation and Causation: The Effect of “Entrepreneurial Experience” and “Market Uncertainty”